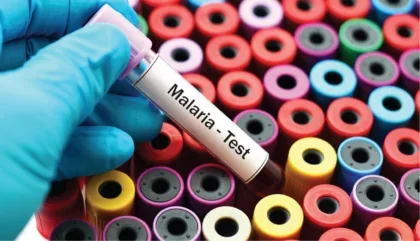
Malaria is a disease that has plagued humanity as far back as ancient Egyptian times. And while, as of December 2024, Egypt has officially been granted malaria-free status, the mosquito-borne infection still claims more than half a million lives each year. Globally, we are still far from having a handle on it, particularly in low-resource areas where effective diagnostic and control measures are hardest to implement.
The first references to malaria date back to the fourth and fifth centuries, but our first real understanding of it came in the late 19th century, when the introduction of Romanowsky stains, such as Giemsa, enabled the microscopic identification of malaria parasites in blood smears, establishing a diagnostic standard that remains in use today.
While this technique has become a cornerstone of malaria diagnosis, it faces significant challenges in endemic regions, largely due to a lack of skilled staff who are capable of conducting the process, which involves not only preparing blood films but also accurately detecting parasites, distinguishing species and avoiding misidentifications.
“The main point here is that there’s a lack of expertise in general; there aren’t enough specialists to diagnose cases at the required level, nor the means to train them,” explains Roxanne Rees- Channer, a malaria researcher at the Hospital for Tropical Diseases at UCLH.
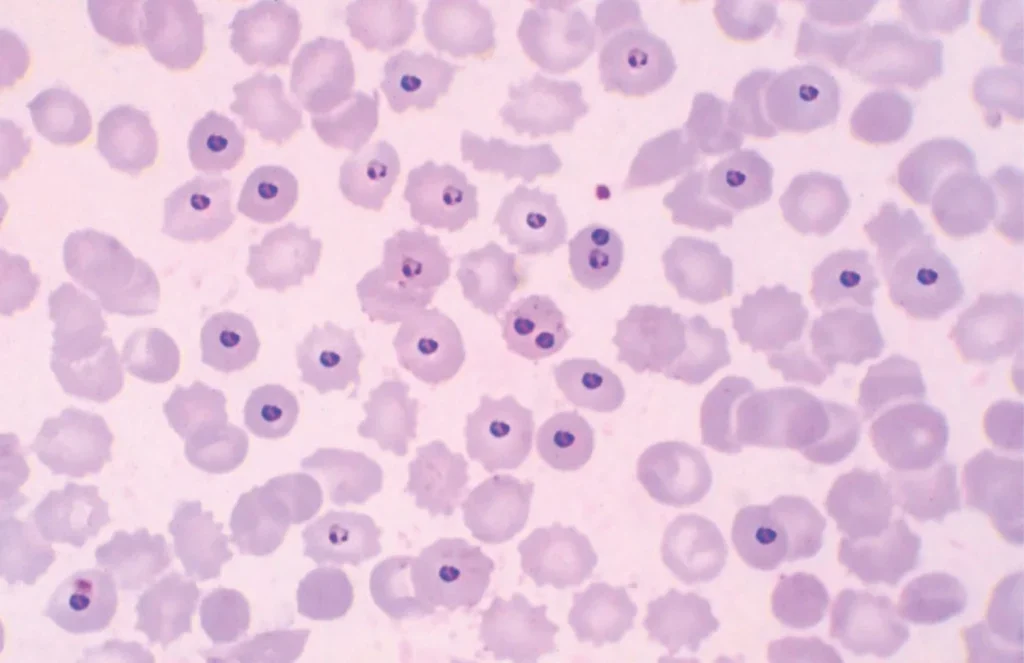
“There are established levels for microscopist expertise, and it’s rare to find a Level 1 microscopist in low-resource settings. Then you’ve got the fatigue issue. Imagine being in an area where there’s a lot of malaria where it takes 30 minutes to read a single slide. If you’re reading slide after slide all day, you’ll inevitably start making mistakes.”
Plus, on the technical side, microscope maintenance is often inadequate in low-resource countries compared to wealthier nations where funding allows for regular upkeep. “In addition, if an experienced technician leaves to work elsewhere, it takes a long time to train a replacement,” Rees- Channer adds.
Given these challenges, other malaria diagnostic techniques have emerged over time, although none is a silver bullet. Molecular diagnostics, such as PCR and loop-mediated isothermal amplification (LAMP), for example, are more sensitive and specific than microscopy, but they are also more expensive and not widely available in most malaria-endemic regions. As Rees-Channer summarises, “These techniques are brilliant but we’re not able to get them to the places where they really need them.”
In addition, there are malaria rapid diagnostic tests (RDTs), which are very affordable – often less than $1 per test. “However, they are less effective at identifying anything beyond Plasmodium falciparum [the deadliest malaria parasite and the most prevalent on the African continent]. They’re also not quantitative – you can’t measure infection intensity,” Rees-Channer notes.
Some Plasmodium falciparum parasites, particularly in Africa, have also developed gene deletions that render them undetectable by certain RDTs. “This means you could get a false-negative result when using an RDT. So, there’s a need for new diagnostics to compensate for these issues. It’s a multifactorial and complex problem.”
It is also one that Rees-Channer is determined to contribute to solving. “I’ve been in malaria research for a while, and I’m very aware of the challenges across every branch of diagnosis. Diagnostics require a multi-pronged approach because it’s so complicated. No single method will ever be perfect, but I was keen to work on something that could fill a hole somewhere or resolve a specific problem,” she explains.
AI vs expert microscopists
Motivated by the potential of AI to revolutionise diagnostics, Rees-Channer joined an international team of researchers exploring its applications in the malaria field. It is one of many similar projects around the world focused on using AI to enhance the accuracy of diagnostic methods and reduce the burden on healthcare workers.
Their study, which was carried out at UCLH and published in Frontiers in Malaria in 2023, evaluated whether a fully automated system – combining AI detection software with an automated microscope – could diagnose malaria with clinically useful accuracy. The goal was to compare this system with the gold standard: manual microscopy.
“There are established levels for microscopist expertise, and it’s rare to find a Level 1 microscopist in low-resource settings.”
The researchers analysed over 1,200 blood samples from travellers returning to the UK from malaria-endemic regions, testing the accuracy of the AI and automated microscope system in a true clinical setting under ideal conditions. Using manual light microscopy, 113 samples were identified as positive for malaria, while the AI system correctly diagnosed 99 positive cases, achieving an 88% accuracy rate.
“It’s a pretty good number by comparison to actual conventional expert microscopy,” Rees- Channer notes. The AI system also offers a significant advantage when it comes to speed. Conventional microscopy requires a technician to scan blood smears manually, identify species and count parasites – a process that takes at least 30 minutes per sample and demands extensive training.
It can take months for a technician to become proficient and even longer to handle complex cases, such as identifying a single parasite in a field of view. By contrast, the AI system simplifies the process: technicians only need to load the slide, and the automated microscope manages the diagnosis with pre-trained software.
That said, the initial AI training process itself was extensive and required several different stages and thousands of thousands of slides to teach the system to identify malaria parasites. Once the initial training stage, which took place in Seattle, where the software was designed, was complete, the team at UCLH were called upon to help before receiving the microscope.
“AI is just one prong in the arsenal. But the fact that it’s not been worked on for very long and is already working well is very impressive.”
“We were sent a load of records of files full of examples of samples that the device had read out and then we had to confirm whether the microscope was right or not. So our expert microscopists spent a period of time looking over these tiles, which gave the software creators the final layer they needed,” Rees- Channer recalls. “It was a complicated process before we even got the device.”
A valuable support tool
The automated system achieved an 88% accuracy rate but also falsely identified 122 samples as positive, which could lead to unnecessary anti-malarial treatment, so there is clearly significant potential for improvement.
“It’s a step on the road; it’s not the endpoint,” Rees- Channer explains. “AI improves as it’s trained, so the more data it processes, the better it gets. It performed particularly well with P. falciparum, which is great.
With more slides and more images, including false positives, true negatives and true positives, the system’s accuracy will only continue to improve.” Clinical trials, like the one conducted at UCLH, are critical to pinpoint areas for refinement. “We’re moving in the right direction, but there’s still work to be done,” she adds.
While not a replacement for human expertise, the AI system could prove to be a valuable support tool, particularly in overwhelmed labs in remote areas. It can oversee initial slide readings, reducing the workload for technicians, although samples would still potentially have to be sent to specialists for confirmation. “It’s a support guide rather than a final result,” Rees-Channer stresses.
One prong in the arsenal
Looking ahead, Rees-Channer is no longer working on AI-assisted malaria diagnostics, but she does remain deeply involved in malaria research. Her current research focuses on antibody responses to single infections over time, exploring different transmission loci. When asked about the future of malaria innovations, she once again emphasises the need for a multi-pronged approach. “Tackling malaria has always been multifactorial,” she says. “The more strategies we have, the closer we’ll get to solving the problem, and it’s going to vary case by case.”
AI-powered diagnostics is just one such tool. Another critical area is the detection of drugresistance markers, vital in regions where resistance to treatment is a growing issue. Alongside these, advancements in malaria vaccines, vector control strategies and improved drug therapies – especially those with fewer side effects – are essential.
“AI diagnostics is just one prong in the arsenal,” Rees-Channer adds. “But the fact that it’s not been worked on for very long and is already working well is very impressive.”