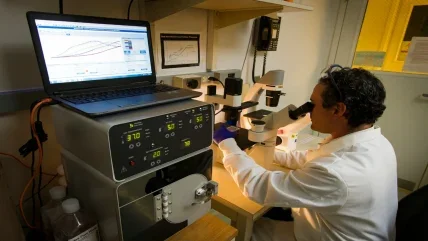
An efficient workflow, combined with traceability, optimal staff usage and, eventually, lowered costs, are the primary requirements of a microbiology laboratory. Automation is an essential prerequisite towards achieving these goals. At Biopole 66, workflow challenges stemmed from a variety of issues. There was a need to merge three separate laboratories with different structures into one entity. “We had three separate labs in the county, and each had been equipped by a different supplier. There were 28 technicians forming three teams of microbiologists, spread over the three labs. In April 2017, the decision was made to create a single structure,” explains Dr Phillipe Schlouch, medical biologist and director at Inovie Group.
Dr Phillipe Schlouch, Inovie Group
Challenges were experienced due to the lack of a smart incubator and the requirement of traceability due to sample volumes. “Each laboratory had an automatic inoculator but no smart incubator. Given the number of specimens that we handled, we wanted to improve process standardisation and the incubation times, as well as enhance the traceability of the samples,” says Dr Marie-France Aran, another of Inovie Group’s medical biologists.
At a more practical level, there were issues related to incubation, plate reading and colony picking. According to Julie Comas, the laboratory technician, the lab had an automatic inoculator and an external incubator, and used a manual batch-reading system that did not allow the control of the incubation period. In addition, the inconsistent quality of the streaking often resulted in colonies that could not be effectively picked.
Effective use of staff time was yet another requirement. According to Schlouch, the idea was to prevent the trained technicians from performing routine, low value work like sorting and handling samples, and instead, get them to perform more important tasks like reading and interpreting cultures.
The proof is in the results
In its quest for a comprehensive solution to improve its workflow, Biopole 66 selected the BD Kiestra Work Cell Automation (WCA) and Urine Culture App (UCA). This is a standardised, scalable and automated solution for inoculation, incubation, plate reading and acceleration of follow-up testing. The BD Kiestra UCA uses sophisticated, artificial intelligence techniques based on decision flowcharts and algorithms to analyse images of urine culture plates. The automated and semi-automated functionalities allow the efficient handling and traceability of all samples. The UCA also allows for the release of plates with non-significant growth for disposal. Only plates with significant culture growth are analysed for follow-up work.
A whole range of benefits has accrued upon the commissioning of the BD Kiestra solution at Biopole 66. After five months of use, the system has helped to streamline workflows and automate low-added-value tasks such as the sorting and handling of plates. In addition, the samples can be accurately tracked, resulting in a high level of traceability. “The lab now successfully inoculates over 80% of its samples, allowing them to be standardised and optimised. We are now able to provide accurate responses to clinician queries,” says Aran.
The BD Kiestra UCA was set up by comparing the practices and standards of the three labs that were being merged. Technical data, patient demographics and medical data were consolidated and integrated to create final operational rules for the BD Kiestra UCA. The unique algorithm allows for the efficient management of early growth, or growth within 11 hours. The plates are read by the BD Kiestra UCA after two, 11 and 18 hours and if the maximum countable level (105 bacteria) is reached after 11 hours, the plate can be processed immediately to initiate follow-up work. “This allows us to save seven hours for the reading alone and, in terms of the work done in a day, we can save approximately one half-day or even one full day’s work,” explains Dr Laurent Bergès, medical biologist and director at Biopole 66.
The solution has also enabled efficient management of personnel, with the staff now spending more time in added value tasks like following up the outputs of the decision-making algorithms. Summing up the overall increase in efficiency, Schlouch says, “The end result of this project is that we now handle larger workloads and offer much higher quality.”